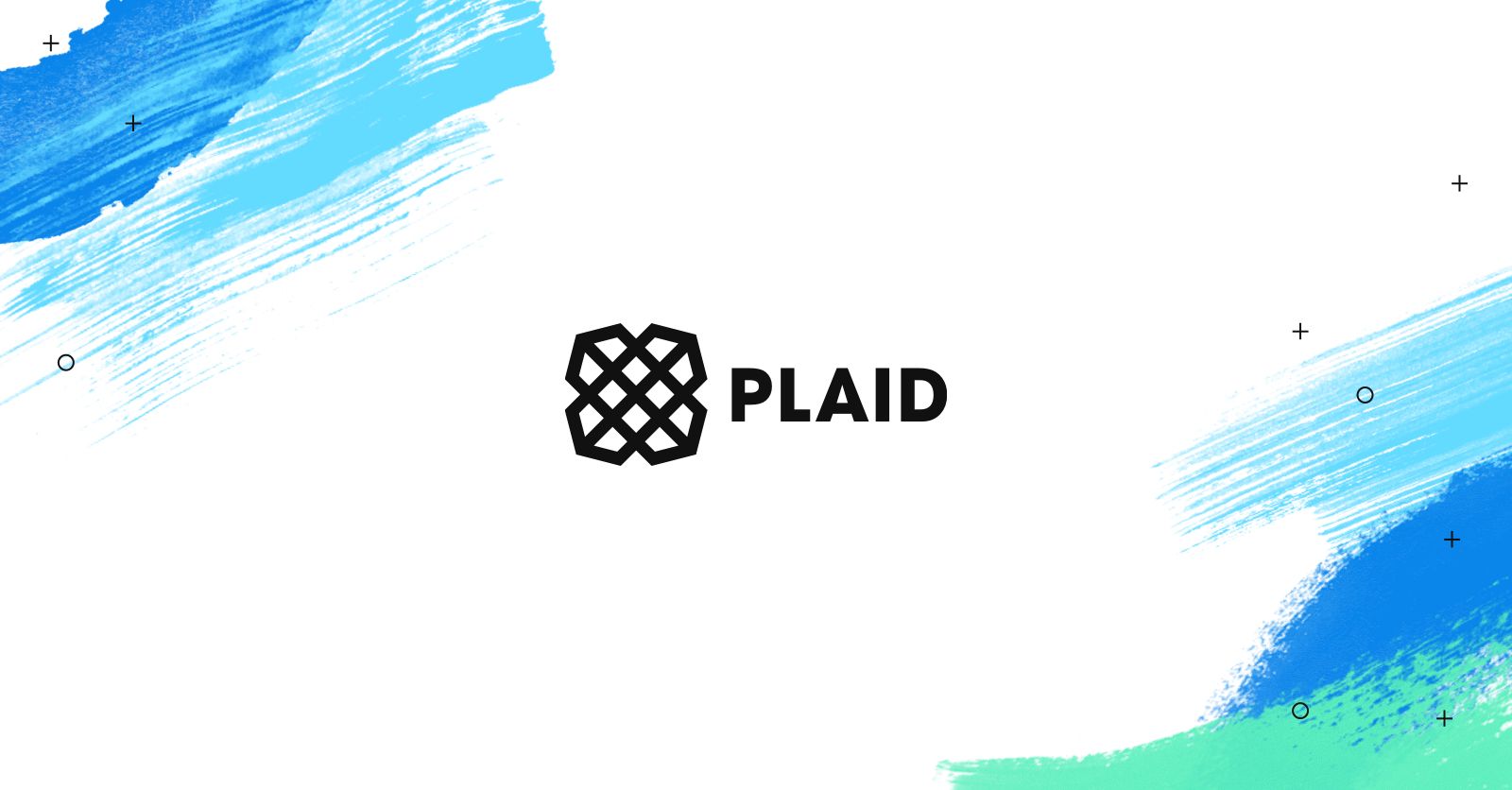
Plaid: The Backbone of Finance
Plaid is a wonderfully crafted, and beautifully executed service that acts as the backbone for some of the largest FinTech companies of this decade. Their portfolio of API-driven solutions, materially reduce friction and allow FinTech companies to better connect with their clients. Odds are that if you've used any modern financial application in the past few years, you've probably used a service built on Plaid's infrastructure without even knowing it.
Plaid has a suite of products that work together to solve common issues seen across various applications of financial technology. The graphic below illustrates Plaid's current product offerings and how they align to a range of use cases in finance. Here's a breakdown of their six products:
- Transactions - Access detailed transaction history and balance data
- Auth - Instantly authenticate bank accounts for ACH and EFT payments
- Identity - Verify users’ identities and reduce fraud
- Balance - Verify real-time account balances
- Assets - Verify borrowers’ assets straight from the source
- Income - Understand income and verify employment
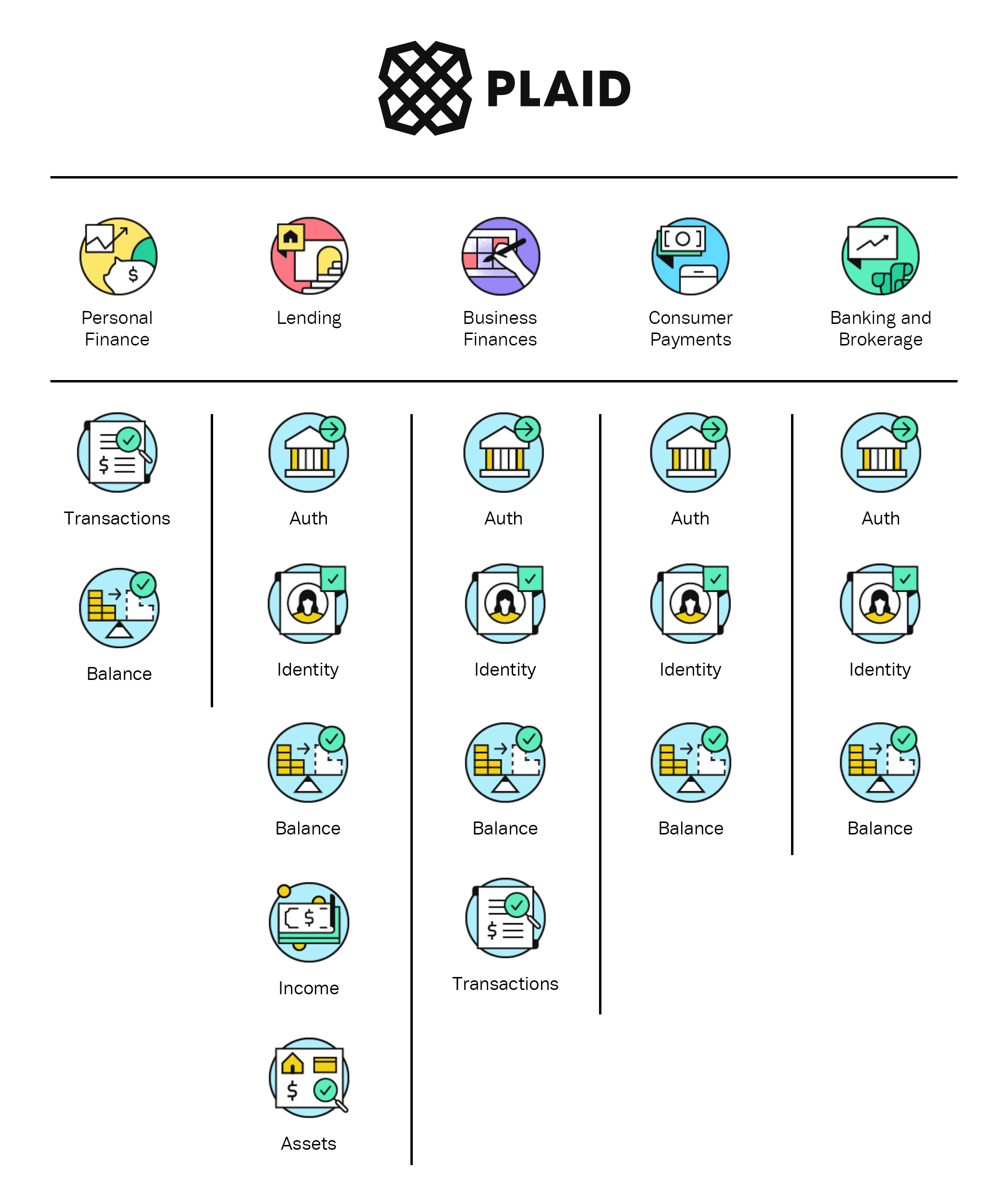
One thing that Plaid does phenomenally well (especially following their rebranding effort) is paint a very clear picture of how each product solves a real problem in finance: issue, solution. It grounds the complexity of FinTech into palatable solutions that drive benefit and impact.
Finance is not "One Size Fits All"
Plaid's product line is comprehensive in its ability to solve a range of use cases. However, there is a subtle lack of specificity to how it solves those problems. More specifically, financial products and services are all designed with a specific consumer in mind - mortgages are for homes, 529 savings plans are for parents saving for their children, IRA's are for everyone who wants to retire, etc.
In the era of modern FinTech, where face-to-face with a financial planner isn't as valued as it was in the past decades, there is still a need for personalization. And in a market of increasing offerings, there is heightened sensitivity to matching consumers with what suits them best.
Issue: Finance is not "one size fits all". There are a host of products and services that support a range of consumer and enterprise financial applications. However, the benefit to clients can be hindered by too large a portfolio of services or offerings. It can also strain customer care, sales, and product teams who are trying to match the right product to the right customer.
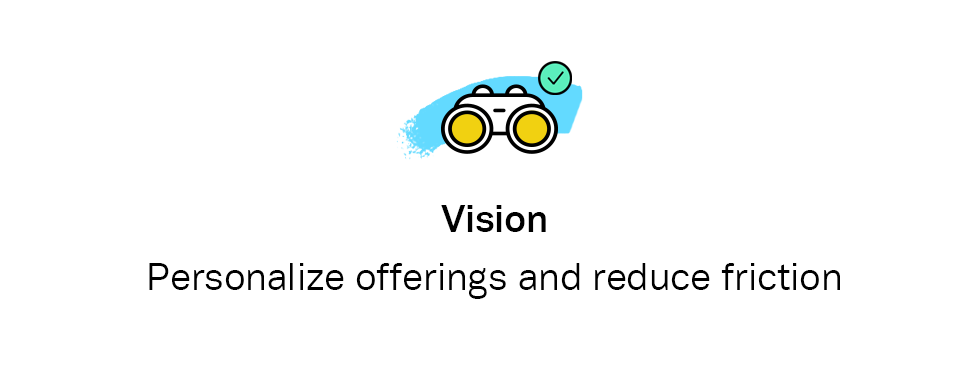
Solution: Plaid derives, prepares, and collates relevant demographic, socioeconomic, and psychographic detail to allow for detailed segmentation of consumers to better target offerings, services, and overall reduce friction in any range of financial offerings.
Note: This isn't a real product of Plaid, just a mockup of one.
Just like census information is used for better planning, targeting, and forecasting of government assistance programs, knowing your client is an easy first step to unlocking a range of customized offerings that can lead to better user retention, higher product utilization, and fewer support requests. Making financial products more targeted and more available leaves everyone better off.
Demographics:
- Age: knowing how old a client is, even if it's just a range, can be immensely useful in building risk profiles for investments, creating time horizons, and understanding financial priorities.
- Life Stage: As with age, understanding the life stage a client is in can help determine priorities and what products or services are most relevant.
- Education: One of the biggest predictors of creditworthiness and wealth, simple classification of clients by education can help make better lending decisions and promote the usage of correctly targeted products.
- Relationship Status/ Family: While seemingly personal, this information is a good indicator of the types of financial products a client might need, including 529 college savings plans for kids, mortgages for new homeowners, IRA's for new graduates, etc. This can also help to approximate tax liabilities in joint versus individual filings, dependents, etc.
- Profession: Although Plaid has a host of products that can determine income and the expected consistency of a paycheck, understanding a client's profession, even at a general level can help piece together risk profiles for loans, or otherwise recommend relevant products (e.g. recommending a 401(k) to a food service employee is poor targeting).
- Lifestyle: Urban, suburban, and rural living are all representative of very different ways of living. Using historical and actual addresses can help build better risk profiles, product targeting, and support channels to meet varying lifestyle's.
Socioeconomic Factors:
- Income: Very easily derived from other Plaid products, a client's income might as well be one of the driving factors of creditworthiness.
- Education: As with the demographic classification, education plays a role in income, occupation, and even likelihood of home ownership.
- Occupation: Very similar to profession as described above; used for better risk profiles and recommending relevant products and services
- Wealth: Derived from Plaid's other products, getting a holistic view of assets (especially over time), combined with age, marital status, family size, occupation, and education are all predictors for wealth attainment. This can be used to target products that provide an ability to meet emergencies (liquid assets), absorb economic shocks (sustainable investments/ lifestyle), or provide the means to live comfortably (retirement planning).
Psychographic Segmentation:
While commonly associated with political campaigns, psychographic segmentation is a powerful tool, though difficult to derive, to segment clients based on their activities, interests, and opinions (AIO variables). In marketing, onboarding, and retention, correct targeting could mean the difference between lifelong customers and high attrition rates.
The caveat is that collecting psychographic information is a careful process. Deriving it can be difficult, especially without skirting moral boundaries of data analysis. Cambridge Analytica is a great example of a company that provides psychographic segmentation, but they've done it in a scrupulous manner that drew harsh scrutiny.
On a simpler level, most psychographic segmentation boils down to an alignment of personality traits in the "five factor model":
- Openness to experience (inventive/curious vs. consistent/cautious)
- Conscientiousness (efficient/organized vs. easy-going/careless)
- Extraversion (outgoing/energetic vs. solitary/reserved)
- Agreeableness (friendly/compassionate vs. challenging/detached)
- Neuroticism (sensitive/nervous vs. secure/confident)
While I won't question the difficulty of gathering this information (accurately), especially without asking or taking a quiz, there are opportunities to extract and analyze data (with permission) to help tailor offerings. For example, a client that allows a Plaid API to scrape their Facebook, twitter, and Instagram profile might be able to build a simple profile based on their activity. Someone who is young and "curious" might be more receptive to new investment opportunities with a brokerage account, or may be more likely to use a nontraditional mortgage product.
This is just an example. Psychographic segmentation should be a long term goal that builds on the segmentation capabilities of demographic and socioeconomic factors as described above. In many respects, it can be considered a form a micro-segmentation - allowing FinTech companies to dial in their targeting efforts even better between clients who may look identical on paper (demographically), but react very differently based upon their personalities.
Plaid "Vision" - Use Cases
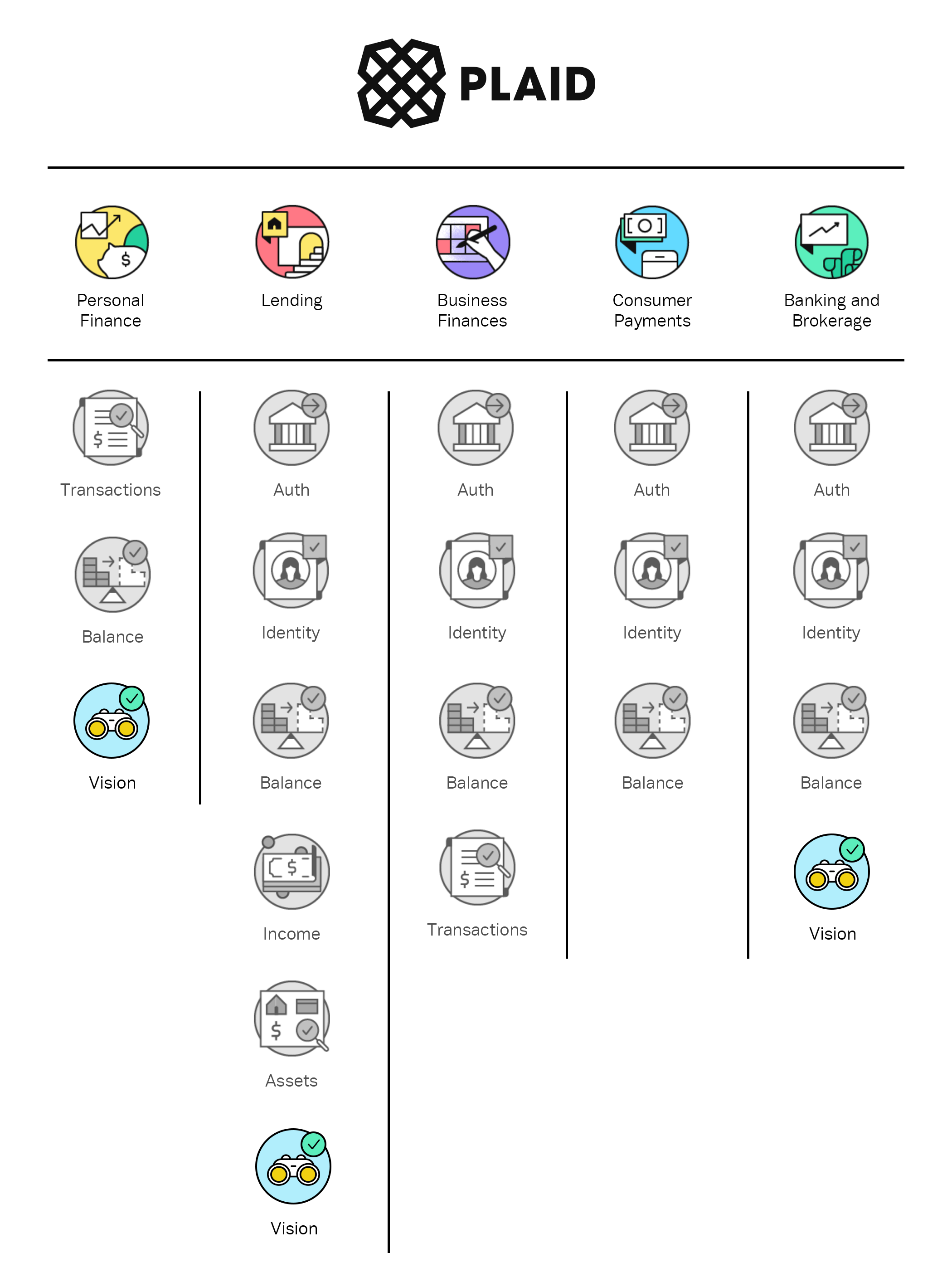
At its core, Vision provides deeper insights into the clients utilizing various financial products, which make it a great addition to personal finance, lending, and banking/ brokerage applications. In the sections below, we'll do a smaller deep dive on how each use case can benefit from the deeper insight and vision of this product.
Personal Finance
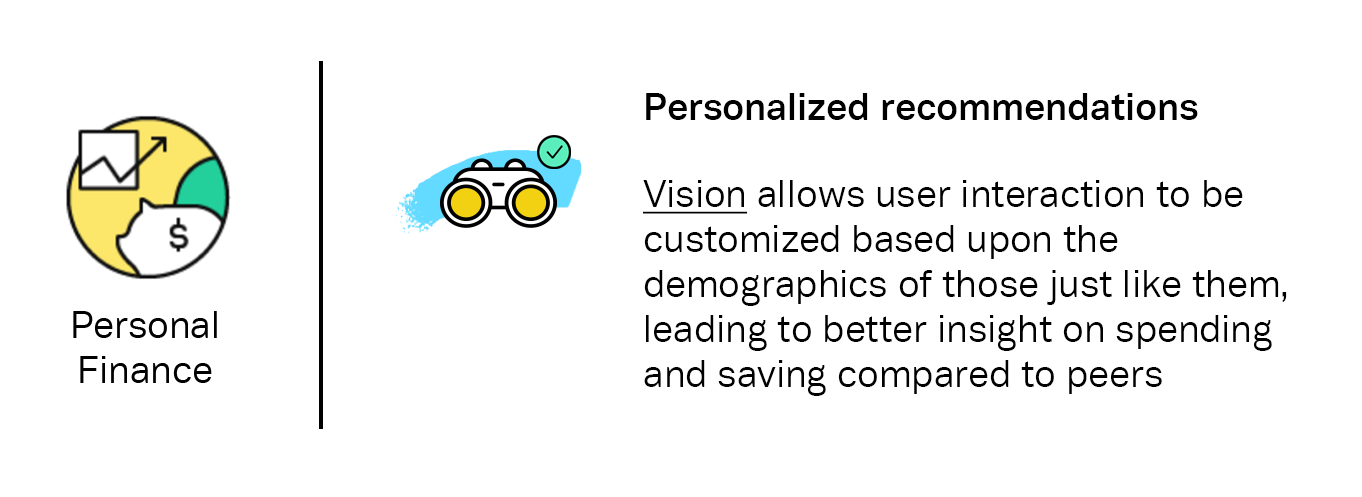
Personal finance has historically, always been personal. While there's nothing wrong with this, the socialization of finance has had some interesting (and largely positive) effects that work in the benefit of the consumer.
For example, you'd be surprised by the amount of articles explaining how much you should have saved by XYZ age. Everyone wants to know how they stack up against those like them. Am I financially better off than those my age? Am I saving more than my age group? Am I spending too much money on restaurants compared to others like me? Am I spending more money online shopping than others like me?
There's an almost limitless combination of segmentation you can provide by matching personal finance habits with the anonymously segmented metrics from demographically similar clients. And the best part, this isn't new. Companies like Mint or CreditKarma have plenty of "insights" littered throughout their applications allowing you to compare your spending or credit score to others like you.
Lending
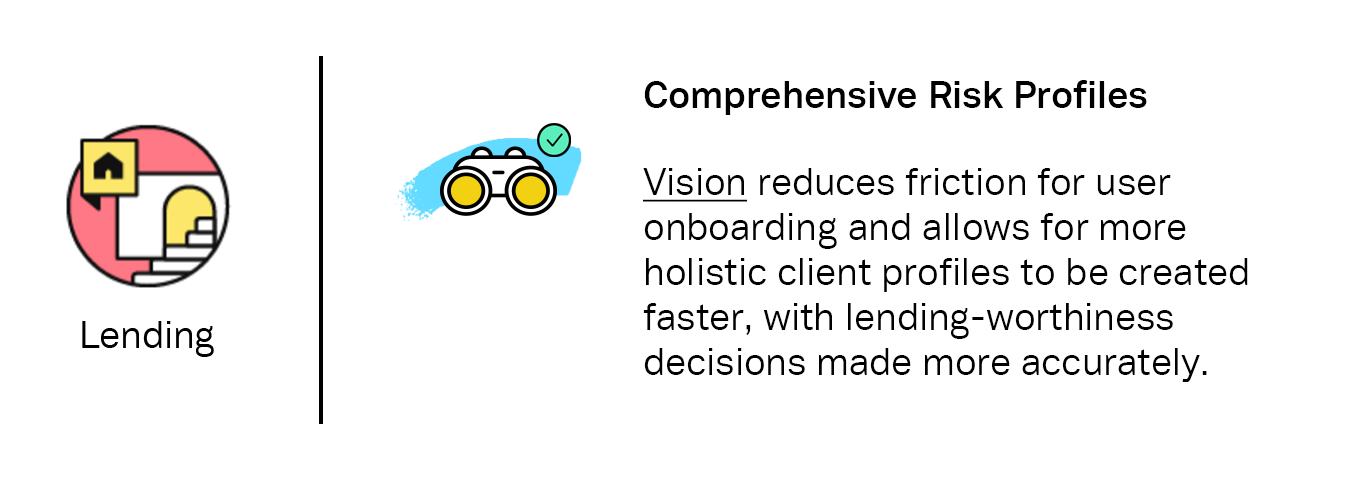
The practice of lending has always been grounded in the creditworthiness of lenders and the risk associated with certain arrangements. While a users credit score is a powerful number that takes into account many financial metrics, it's inherently limited in some applications. One such application is lending for Millennial's/ Gen Z whom may not have well established credit profiles. How else do you determine the default risk of a loan with limited information?
Turns out the Government solves this problem every year when Federal Student Loans are determined, and they've figured out that loan-delinquency rates differ sharply across demographic groups.
Adding demographic information to lending profiles can help better calculate risk and default rates. Since we know that demographics are good predictors of credit worthiness, especially when looking at "good" loans, like federal student loans, or scenarios where applicants do not have largely established credit profiles, utilizing demographics can help piece together a more holistic profile to make better decisions.
This also has the added benefit of reducing friction since you don't have to ask for additional information that may help users get through setup or on-boarding quicker and allow lending companies to provide decisions sooner.
Banking and Brokerage
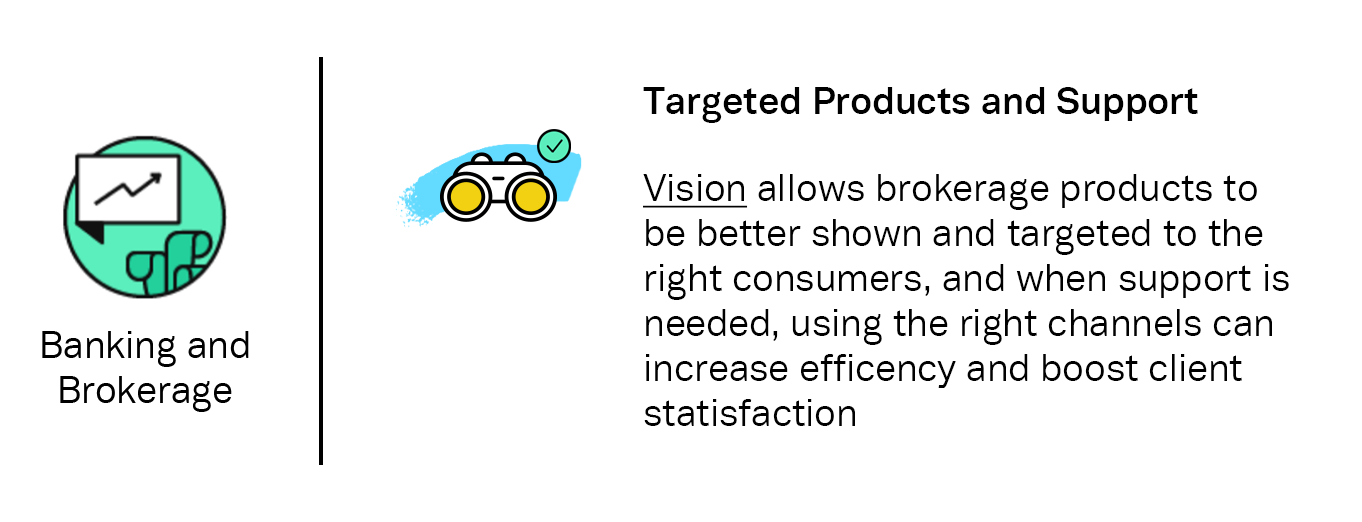
Banking and brokerage is on the forefront of financial modernization: we've made leaps and bounds in democratizing access to markets and bank accounts. There's still the question of education, or financial literacy in general, but you can imagine that if you're putting the right products and services in front of the right consumers you'll get better conversions.
Add demographics to client profiles makes it easy for product placement, especially in the brokerage space. For example: IRA's are great retirement vehicles and should be encouraged to those who are perhaps opening their first account and 529 college savings plan are great for new parents. There's a web of combinations that allow better targeting here that signup screens with a range of products and services that might not be well targeted and may serve to confuse rather than help.
Finally, as an auxiliary benefit, you now have an ability to provide support options that make most sense to the consumer you're targeting. For example, Millennial's prefer self-service, messaging (texting) over calls, want fast help, and an experience that is personalized to them. Think about how support happens in the Uber app versus Edward Jones. Which is more friendly for Millennial's? Gen X? Baby Boomers? If you knew how your client wanted to be helped, and in a manner they would find most helpful, you could redeploy human capital and technology assets to more efficiently use time, resources, and money.
Member discussion: